Accurate and scalable social recomendation using mixed-membership stochastic block models
Times cited: 60
Godoy-Lorite, A, Guimera, R, Moore, C, Sales-Pardo, M.
Proc. Natl. Acad. Sci. USA
113 (50)
,
14207
-14212
(2016).
Recommendation systems are designed to predict users’ preferences and provide them with recommendations for items such as books or movies that suit their needs. Recent developments show that some probabilistic models for user preferences yield better predictions than latent feature models such as matrix factorization. However, it has not been possible to use them in real-world datasets because they are not computationally efficient. We have developed a rigorous probabilistic model that outperforms leading approaches for recommendation and whose parameters can be fitted efficiently with an algorithm whose running time scales linearly with the size of the dataset. This model and inference algorithm open the door to more approaches to recommendation and to other problems where matrix factorization is currently used.
Media coverage
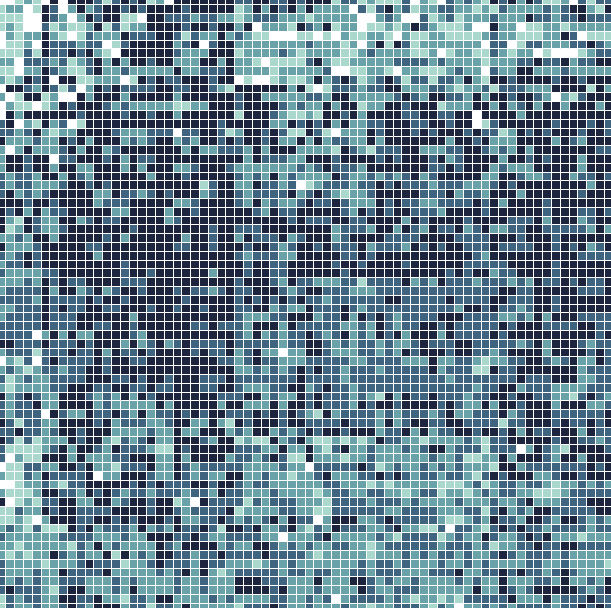