Fundamental limits to learning closed-form mathematical models from data
Times cited: 17
Fajardo-Fontiveros, O, Reichardt, I, De Los Ríos, HR, Duch, J, Sales-Pardo, M, Guimerà, R.
Nat. Comm.
14
,
1043
(2023).
Given a finite and noisy dataset generated with a closed-form mathematical model, when is it possible to learn the true generating model from the data alone? This is the question we investigate here. We show that this model-learning problem displays a transition from a low-noise phase in which the true model can be learned, to a phase in which the observation noise is too high for the true model to be learned by any method. Both in the low-noise phase and in the high-noise phase, probabilistic model selection leads to optimal generalization to unseen data. This is in contrast to standard machine learning approaches, including artificial neural networks, which in this particular problem are limited, in the low-noise phase, by their ability to interpolate. In the transition region between the learnable and unlearnable phases, generalization is hard for all approaches including probabilistic model selection.
Media coverage
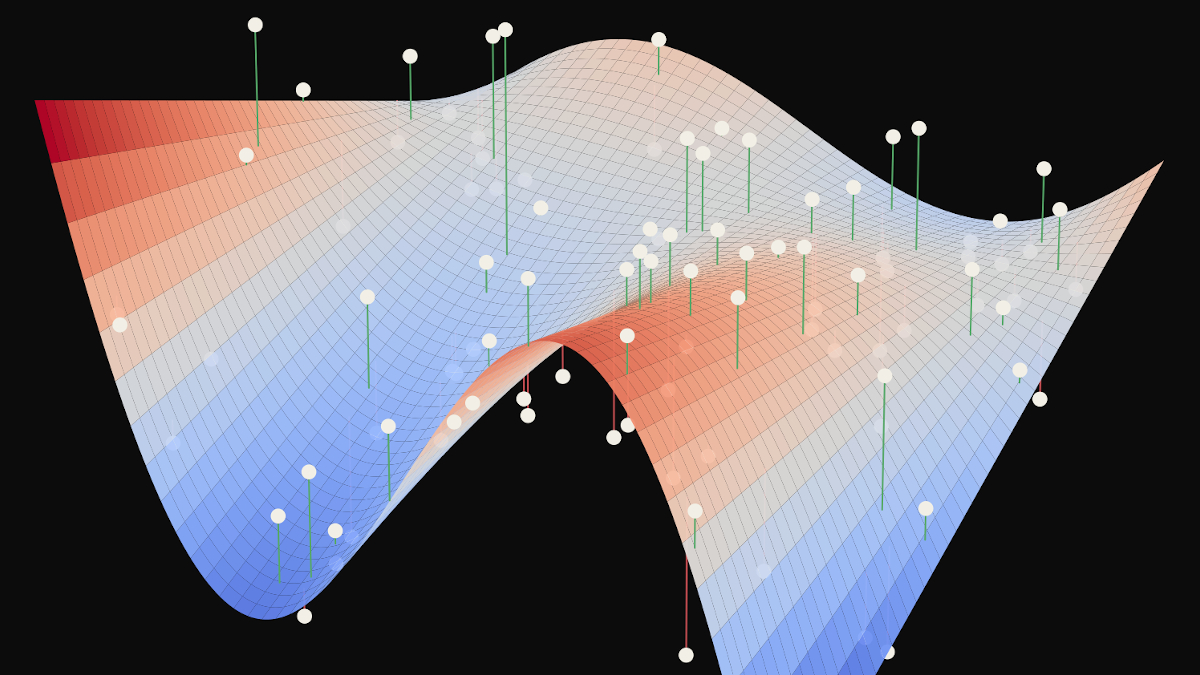